The Ultimate Guide to Old Yorkie Care: Answering Your Top 10 Questions
Yorkies, with their charming personalities and luxurious coats, have stolen the hearts of many dog lovers. But as they age, these once spunky pups can face some unique challenges. This comprehensive guide will answer your top 10 questions about Old Yorkie care, providing you with the knowledge and resources you need to ensure your senior Yorkie lives a long and happy life.
From understanding their changing needs to providing the best possible care, we’ll delve into everything from nutrition and exercise to common health concerns and end-of-life care.
1. What Are the Signs of Aging in a Yorkie?
As Yorkies age, their bodies undergo natural changes that can impact their appearance, behavior, and overall health. Just like humans, senior Yorkies may experience a slower pace of life, some physical limitations, and changes in their sleep and eating habits. Recognizing these signs can help you provide the best care for your aging companion.
Here are some common signs of aging in a Yorkie:
- Decreased Activity Level: Your Yorkie may become less interested in playing, going for walks, or engaging in other activities they once enjoyed.
- Changes in Sleep Patterns: Senior Yorkies often sleep more than younger dogs, and their sleep may be fragmented.
- Weight Changes: Some Yorkies may gain weight as they age, while others may lose weight due to decreased appetite or digestive issues.
- Cognitive Decline: Similar to humans, Yorkies can experience cognitive decline, leading to confusion, disorientation, or anxiety.
- Graying Muzzle and Coat: While not a health concern, graying around the muzzle and in the coat is a common sign of aging.
- Decreased Muscle Mass: As Yorkies age, their muscles may atrophy, resulting in decreased strength and mobility.
- Changes in Vision and Hearing: Senior Yorkies may experience vision and hearing loss, which can affect their ability to navigate and respond to their environment.
- Increased Thirst and Urination: Age-related changes in kidney function can lead to increased thirst and urination.
- Joint Pain and Stiffness: Arthritis is a common ailment in older dogs, causing joint pain, stiffness, and difficulty moving.
If you notice any significant changes in your Yorkie’s behavior or physical appearance, it’s essential to schedule a checkup with your veterinarian. Early detection and treatment of any health issues can help improve your Yorkie’s quality of life.
2. How Can I Make My Old Yorkie More Comfortable?
As your Yorkie ages, they may require special accommodations to help them remain comfortable and mobile. Making some simple adjustments to your home environment can significantly improve your Yorkie’s quality of life.
Here are some tips to make your old Yorkie more comfortable:
- Soft Bedding: Provide your Yorkie with a soft and comfortable bed. You can consider a memory foam bed for added support.
- Ramps and Steps: If your Yorkie has difficulty navigating stairs, invest in ramps or steps to help them get on and off furniture or beds.
- Non-Slip Surfaces: Use non-slip mats or rugs in areas where your Yorkie walks to prevent slipping and falls.
- Elevated Food and Water Bowls: Older Yorkies may have difficulty bending down to eat and drink. Using elevated bowls can help them stay comfortable.
- Regular Grooming: Grooming your Yorkie’s coat regularly is essential for maintaining their comfort. Long nails can cause pain and difficulty walking, and mats can cause discomfort.
- Supportive Harness or Sling: If your Yorkie has difficulty walking, a supportive harness or sling can help you assist them with movement.
- Warmth: Older dogs may be more sensitive to cold temperatures. Keep your Yorkie warm with a cozy blanket or sweater.
- Create a Calming Environment: A calm and quiet environment can help reduce stress and anxiety for your aging Yorkie. Limit noise and distractions.
- Regular Veterinary Checkups: Regular veterinary checkups are crucial for monitoring your Yorkie’s health and catching any potential problems early on.
By making these adjustments, you can create a safe and comfortable environment for your aging Yorkie, allowing them to enjoy their golden years.
3. What Should I Feed My Old Yorkie?
As Yorkies age, their dietary needs change. Providing them with a balanced and nutritious diet can help maintain their health, energy levels, and overall well-being.
Here are some key considerations for feeding your old Yorkie:
- High-Quality Senior Dog Food: Choose a senior dog food specifically formulated for older dogs. These formulas are designed to meet their changing nutritional needs, often containing higher levels of glucosamine and chondroitin for joint health, and lower calorie counts to prevent weight gain.
- Smaller Meals More Frequently: Older Yorkies may have slower digestive systems, so it’s often better to feed them smaller meals more frequently throughout the day rather than one large meal.
- Fresh Water: Ensure your Yorkie has access to fresh water at all times. Older dogs can be more prone to dehydration.
- Dental Care: Dental health is vital for older dogs. Brush your Yorkie’s teeth regularly, and consider dental chews or treats to help maintain good oral hygiene.
- Avoid Overfeeding: Overfeeding can lead to weight gain, which can put extra stress on joints and organs. Consult your veterinarian for appropriate feeding guidelines.
- Consider Supplements: Your veterinarian may recommend supplements to address specific health concerns, such as joint pain or cognitive decline.
Feeding your old Yorkie a healthy diet can help them maintain their strength, energy, and quality of life. Consult with your veterinarian to create a personalized feeding plan that meets your Yorkie’s individual needs.
4. How Much Exercise Does an Old Yorkie Need?
While older Yorkies may require less vigorous exercise, it’s still essential for maintaining their mobility, flexibility, and overall health. However, you’ll need to adjust their exercise routine to accommodate their age and physical limitations.
Here are some tips for exercising your old Yorkie:
- Short, Gentle Walks: Instead of long walks, opt for shorter, more frequent walks. Choose gentle walking surfaces like grass or pavement.
- Swimming: If your Yorkie enjoys swimming, it’s a great low-impact exercise option. Swimming is gentle on joints and can help maintain muscle strength.
- Indoor Activities: Consider indoor activities like fetch or hide-and-seek to keep your Yorkie mentally and physically stimulated.
- Rest Periods: Allow your Yorkie to rest frequently, especially after exercise. Older dogs may need more time to recover.
- Avoid Overexertion: It’s crucial to avoid overexerting your old Yorkie. Watch for signs of fatigue, such as panting, slowing down, or reluctance to continue.
- Joint Support: Consider joint support supplements for your Yorkie to ease any stiffness or discomfort.
- Monitor for Pain: Pay attention to any signs of pain during or after exercise, such as limping or reluctance to move.
Adjust your Yorkie’s exercise routine to their needs, keeping in mind that gentle movement is key to maintaining their health and well-being. Always consult with your veterinarian before starting any new exercise program.
5. What Are Some Common Health Problems in Old Yorkies?
As Yorkies age, they’re more susceptible to certain health problems. Understanding these potential issues can help you recognize the signs early and seek appropriate care.
Here are some common health problems in old Yorkies:
- Arthritis: Arthritis is a common ailment in older dogs, causing joint pain, stiffness, and difficulty moving.
- Dental Disease: Dental disease can lead to pain, infection, and other health problems. Regular dental care is crucial for older Yorkies.
- Kidney Disease: Kidney disease can occur in older dogs and can lead to increased thirst, urination, and loss of appetite.
- Heart Disease: Heart disease is another common health concern in senior dogs. Symptoms can include coughing, difficulty breathing, and weakness.
- Cancer: Unfortunately, cancer is a possibility in older dogs. Be aware of any lumps, bumps, or unusual changes in your Yorkie’s body.
- Cognitive Decline: Cognitive decline, also known as canine dementia, can affect your Yorkie’s mental capabilities, leading to confusion, disorientation, and anxiety.
- Eye Problems: Eye problems like cataracts, glaucoma, and retinal degeneration can occur in older dogs.
- Hearing Loss: Hearing loss is common in older dogs and can affect their ability to respond to their environment.
Regular veterinary checkups are essential for early detection and treatment of any health problems. If you notice any changes in your Yorkie’s behavior or appearance, don’t hesitate to schedule a checkup.
6. How Can I Help My Old Yorkie Cope With Cognitive Decline?
Cognitive decline, or canine dementia, can affect your Yorkie’s mental capabilities and lead to changes in behavior. While there’s no cure, you can implement strategies to help your Yorkie cope and maintain their quality of life.
Here are some tips for managing cognitive decline in an old Yorkie:
- Create a Routine: Maintain a consistent daily routine with regular feeding times, walks, and playtime. This can help reduce confusion and anxiety.
- Calming Environment: Ensure a calm and quiet environment to minimize stress and confusion. Reduce noise and distractions.
- Mental Stimulation: Engage your Yorkie in mentally stimulating activities, such as puzzles, hide-and-seek games, or interactive toys.
- Physical Activity: Continue to provide gentle exercise to help maintain your Yorkie’s physical and mental well-being.
- Supportive Communication: Speak to your Yorkie in a calm and reassuring tone, using clear and simple language. Provide positive reinforcement and encouragement.
- Consider Dietary Supplements: Consult your veterinarian about supplements that may help support cognitive function.
- Seek Professional Guidance: Don’t hesitate to reach out to your veterinarian or a canine behaviorist for professional guidance and support.
Remember, patience and understanding are key when dealing with cognitive decline in your Yorkie. By providing a supportive and stimulating environment, you can help them live a happy and fulfilling life despite their age.
7. How Can I Make My Old Yorkie’s Last Days Comfortable?
As your Yorkie reaches the end of their life, it’s essential to provide them with love, comfort, and the best possible care. This includes recognizing the signs of approaching end-of-life and making decisions that prioritize their well-being.
Here are some tips for making your Yorkie’s last days comfortable:
- Observe for Signs: Pay close attention to any changes in your Yorkie’s behavior, such as decreased appetite, lethargy, weight loss, increased thirst, or difficulty breathing. These can indicate that their health is declining.
- Quality of Life: Consult with your veterinarian to assess your Yorkie’s quality of life. Consider factors like their ability to eat, drink, walk, and interact with their environment.
- Pain Management: Discuss pain management options with your veterinarian to ensure your Yorkie is comfortable. Medications can help alleviate pain and discomfort.
- Home Care: If your Yorkie’s condition allows, you can provide them with comfort and care at home. This may involve providing a soft bed, warm blankets, and regular cleaning.
- Compassionate Companionship: Spend quality time with your Yorkie, providing them with love, affection, and companionship. Allow them to rest peacefully.
- Difficult Decisions: In some cases, euthanasia may be the most humane option. Discuss this with your veterinarian and make decisions that are in your Yorkie’s best interest.
Saying goodbye to a beloved pet is never easy, but providing them with love and compassion during their final days is a gift they will cherish. Remember, you’re not alone in this journey. Reach out to your veterinarian, family, or support groups for help and guidance.
8. Can Old Yorkies Be Adopted?
Many senior Yorkies find loving homes through adoption. Adoption can be a rewarding experience for both you and the dog. Senior dogs often come with pre-existing medical conditions, but they can also bring years of love and companionship.
Here are some things to consider when adopting an old Yorkie:
- Be Prepared for Medical Needs: Older dogs often have pre-existing health conditions, so be prepared for potential vet bills and ongoing care.
- Patience and Understanding: Senior dogs may have learned behaviors or quirks that might require patience and understanding.
- Lifestyle Compatibility: Ensure your lifestyle is compatible with the needs of a senior dog, such as their exercise requirements and potential for accidents.
- Research Rescues: Look for reputable rescue organizations that specialize in senior dogs. They can provide you with information about the dog’s history and health.
- Meet and Greet: Take the time to meet and interact with the Yorkie before adopting them. This will help you assess their personality and compatibility with your family.
Adopting a senior Yorkie can be a fulfilling experience, giving a loving home to a dog who needs it. Be prepared for the unique challenges and rewards that come with caring for a senior dog.
9. How Can I Prevent My Yorkie From Getting Lost?
Even though your Yorkie may be less active, getting lost can still be a risk. Here are some tips to help prevent your Yorkie from getting lost:
- Microchip: Microchipping is a crucial step in keeping your Yorkie safe. It’s a small, permanent implant that contains your contact information.
- Collar with ID Tags: Make sure your Yorkie wears a collar with clearly visible ID tags that include your name, phone number, and address.
- Leash Laws: Always keep your Yorkie on a leash when walking in public areas. This prevents them from wandering off and getting lost.
- Fenced Yard: Ensure your yard is securely fenced to prevent escapes. Regularly inspect the fence for any holes or weak spots.
- Harness: Consider using a harness in addition to a collar. A harness provides extra security and can prevent your Yorkie from slipping out of their collar.
- Training: Train your Yorkie to come when called. This command can be crucial if they ever get loose.
- Lost Dog Posters: If your Yorkie does get lost, post flyers with their picture and description in your neighborhood and surrounding areas. Contact local animal shelters and rescues.
Taking these precautions can help ensure your Yorkie stays safe and returns home to you. Remember, prevention is key to keeping your Yorkie safe.
10. What are the Costs Associated with Caring for an Old Yorkie?
Caring for a senior Yorkie comes with additional costs, especially as they may require more frequent vet visits and specialized care. It’s wise to budget for these potential expenses.
Here are some of the costs associated with caring for an old Yorkie:
- Veterinary Care: Regular checkups, vaccinations, dental care, and potential treatments for age-related conditions can add up.
- Prescription Medications: Medications for arthritis, heart disease, or other health concerns can be expensive.
- Special Diets: Prescription or specialized senior dog food may be necessary to meet your Yorkie’s changing nutritional needs.
- Supplements: Supplements for joint health, cognitive function, or other health concerns can add to your expenses.
- Grooming: Older Yorkies may require more frequent grooming to manage their coat. This includes brushing, bathing, and nail trimming.
- Home Modifications: You may need to make home modifications to accommodate your Yorkie’s mobility needs, such as ramps or steps.
It’s essential to budget for these costs and ensure you can provide your Yorkie with the best possible care as they age. Consider pet insurance to help manage unexpected expenses.
Table Summarizing Old Yorkie Care
Category | Tips |
---|---|
Comfort | Soft bedding, ramps and steps, non-slip surfaces, elevated bowls, regular grooming, supportive harness or sling, warmth, calming environment, regular veterinary checkups. |
Nutrition | High-quality senior dog food, smaller meals more frequently, fresh water, dental care, avoid overfeeding, consider supplements. |
Exercise | Short, gentle walks, swimming, indoor activities, rest periods, avoid overexertion, joint support, monitor for pain. |
Health | Regular veterinary checkups, understand common health problems, monitor for signs of illness, provide appropriate care and treatment. |
Cognitive Decline | Create a routine, calming environment, mental stimulation, physical activity, supportive communication, consider dietary supplements, seek professional guidance. |
End-of-Life Care | Observe for signs of decline, assess quality of life, manage pain, provide home care, compassionate companionship, discuss euthanasia options. |
Adoption | Be prepared for medical needs, patience and understanding, lifestyle compatibility, research rescues, meet and greet. |
Safety | Microchip, collar with ID tags, leash laws, fenced yard, harness, training, lost dog posters. |
Costs | Veterinary care, prescription medications, special diets, supplements, grooming, home modifications. |
FAQ
Here are some frequently asked questions about Old Yorkie care:
How Long Do Old Yorkies Live?
The average lifespan of a Yorkie is 12 to 15 years, but they can live longer with proper care. As with any dog breed, genetics, lifestyle, and health care play a role in their lifespan. Providing a healthy diet, regular exercise, and preventative veterinary care can help extend their life.
What Are the Best Ways to Keep an Old Yorkie Entertained?
Older Yorkies may have less energy for vigorous play, but they still need mental stimulation. Consider these activities:
- Puzzle Toys: These toys challenge their minds and provide enrichment.
- Interactive Games: Games like hide-and-seek or fetch can keep them engaged.
- Training Sessions: Short training sessions can help keep their minds sharp and reinforce their bonds with you.
- Socialization: Safe and supervised interaction with other dogs can provide mental stimulation and social interaction.
What Are the Signs of a Dying Yorkie?
It’s important to recognize the signs of a dying Yorkie to provide them with compassionate care in their final days. These signs can include:
- Decreased Appetite and Thirst: Loss of interest in food and water is common as their bodies weaken.
- Lethargy: They may become very sleepy and less active.
- Difficulty Breathing: Panting, gasping, or labored breathing are common in their final stages.
- Weight Loss: They may lose muscle mass and become thinner.
- Incontinence: They may have difficulty controlling their bladder or bowels.
- Changes in Behavior: They may become disoriented, confused, or more clingy.
- Weakness: They may have difficulty standing or walking.
Is It Okay to Give My Old Yorkie Human Food?
It’s generally not recommended to give your Yorkie human food. Many human foods are unhealthy for dogs and can lead to digestive problems or other health issues. Stick to a high-quality senior dog food formulated to meet their specific needs.
How Can I Help My Old Yorkie With Arthritis Pain?
Arthritis is a common condition in older Yorkies, and there are ways to manage the pain and discomfort. Consult with your veterinarian for personalized recommendations, which may include:
- Pain Medications: Nonsteroidal anti-inflammatory drugs (NSAIDs) or other pain relievers can help reduce pain.
- Joint Supplements: Glucosamine and chondroitin supplements can support joint health and reduce inflammation.
- Weight Management: Excess weight can put extra stress on joints. Maintain a healthy weight for your Yorkie.
- Physical Therapy: Gentle exercise and physical therapy can help improve mobility and flexibility.
- Acupuncture: Acupuncture can help reduce pain and inflammation.
- Hydrotherapy: Water therapy can provide low-impact exercise and help reduce joint stress.
What Should I Do if My Old Yorkie Has an Accident in the House?
Accidents happen, and older dogs may have difficulty controlling their bladder or bowels. Here’s what to do:
- Clean Up Promptly: Clean up the accident as soon as possible to prevent odors and potential bacterial growth.
- Use an Enzyme Cleaner: Use an enzyme cleaner specifically designed for pet accidents. This will break down the odor and make it less likely for your Yorkie to have another accident in the same spot.
- Consult Your Veterinarian: If accidents become frequent, consult your veterinarian to rule out any underlying medical conditions.
What are some signs that my old Yorkie is not getting enough exercise?
Here are some signs that your old Yorkie may not be getting enough exercise:
- Weight Gain: If your Yorkie is gaining weight despite eating the same amount of food, they may not be burning enough calories.
- Decreased Energy Levels: If your Yorkie seems lethargic, sleeps more than usual, or is less interested in playing, they may not be getting enough exercise.
- Joint Stiffness: Lack of exercise can contribute to joint stiffness and arthritis, making it harder for your Yorkie to move around.
- Behavioral Changes: Your Yorkie may become more anxious, restless, or destructive if they aren’t getting enough physical and mental stimulation.
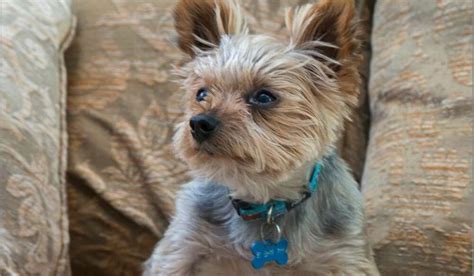